- Contact Email: jacob.leygonie@gmail.com
About Me
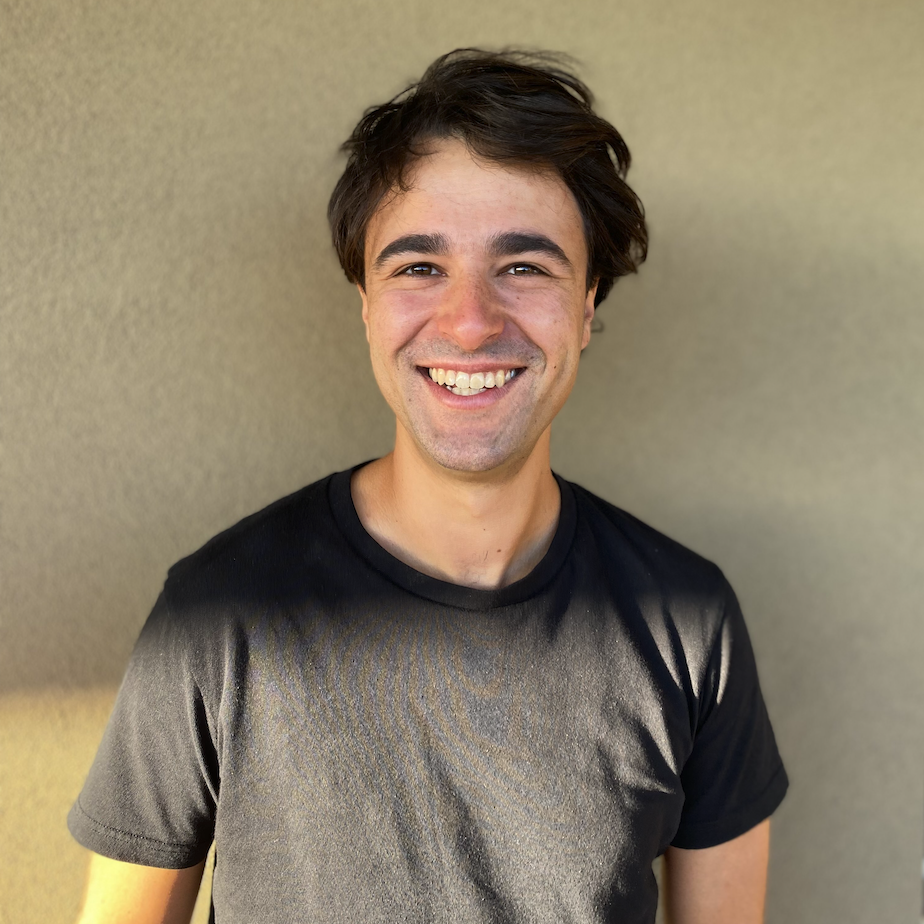
Welcome to my research webpage! I am Jacob Leygonie, a 28 years old French mathematician. My passions include:
- Algebraic Topology and its applications to Data Analysis & Inverse Problems,
- Theory of (non-smooth, non-convex) Optimisation and applications to Deep Learning,
- Optimal Transport, both the theoretical challenges and relations to Deep Learning.
I completed my PhD in March 2022 at the Mathematical Institute of Oxford, where I was a member of the Algebraic Topology group and of the Center for Topological Data Analysis, supervised by Pr. Ulrike Tillmann and Pr. Heather Harrington. Here is my thesis!
After this I started working at Inria Paris with Pr. Steve Oudot as an LMS Early Career Fellow.
Before the PhD, I did a 6 months research internship in Deep Learning at M.I.L.A in Montréal, after completing a BS and MsC in Mathematics and Computer Science at the Ecole Polytechnique in France.
Research Interests
My research lies at the crossroads between Applied Topology, Non-Smooth Optimisation, Optimal Transport and Deep Learning.
a. Computational Geometry and Topology
During my thesis, I studied Persistent Homology (PH), a rapidly growing topological descriptor for data sets such as graphs and point clouds. For a given data set I asked myself two naive yet fundamental questions to know if using PH is relevant:
- Is PH sufficiently discriminative for this data set?
- Can we optimise the parameters of PH for this data set, and can we use it concurrently with other tools, e.g. Machine Learning models?
These practical questions inevitably entail the theoretical problematics I addressed during my PhD:
- What are the objects with the same PH? What are the geometric and topological properties of the pre-image of PH?
- Is there a framework for differential calculus and optimisation adapted to PH?
b. Non-smooth Optimisation
Modern neural networks pipelines involve non-smooth and non-convex objective functions, for which it is key to design reliable optimisation procedures and convergence guarantees.
As I was exploring extensions of standard Differential Geometry to more complex spaces during my thesis, I ended up developping a particular taste for non-smooth Optimization, and I am now investigating its theory and algorithms.
c. Optimal Transport and Deep Learning
Optimal Transport (OT) brings together the beautiful disciplines of probabilities, differential equations and optimisation to solve the problem of transporting the mass of one probability distribution onto another at minimal cost.
I have strong interest for some theoretical properties of the well-known Sinkhorn version of the OT problem, which has efficient implementations and numerous applications.
In addition, the Brenier formulation of OT in terms of partial differential equations has neat relations with Generative Adversarial Networks, which I have been exploring as a way to generate meaningful mappings between real-life datasets.